When financial behemoths Bear Stearns and Lehman Brothers went bankrupt in 2008, the entire U.S. financial sector shuddered, and, for a brief period, it seemed as if they might take down more institutions like them.
For Yi Tang, Ph.D., an assistant professor of finance and business economics in the Fordham Schools of Business, this was evidence that there is data that is not being explored to its full potential.
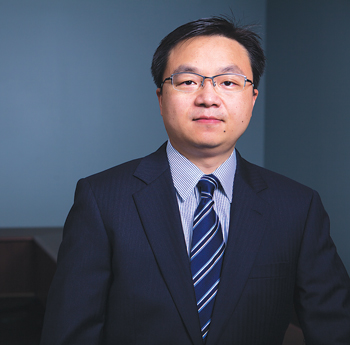
Photo by Bud Glick
How, Tang wondered, could we create a measure that can deliver an early warning system to signal whether aggregate systemic risk in the financial sector presages future economic declines?
The answer to the problem is CATFIN (Catastrophic Risk in the Financial Sector), a measure that captures the aggregate risk-taking by the entire financial sector, and complements bank-specific systemic risk measures by forecasting macroeconomic downturns six months into the future.
The CATFIN measure is detailed in the article “Does Systemic Risk in the Financial Sector Predict Future Economic Downturns?” published in the October 2012 issue of the Review of Financial Studies, which Tang co-wrote with Linda Allen, Ph.D., the William F. Aldinger Chair in Banking and Finance at Baruch College, and Turan Bali, Ph.D., the Robert S. Parker Chair of Business Administration at Georgetown University.
The authors came up with a measurement based on extreme loss from U.S. financial firms. They then used a set of macroeconomic variables such as the Chicago Fed National Activity Index, the gross domestic product (GDP) of the United States, and the National Bureau of Economic Research Recession Indicator, to measure the health of the entire U.S. economy.
Their idea was that extreme losses that show up in those measures would be a red flag indicating that financial instability is on the horizon. Regulators can take preemptive actions and set a more constraining limit or higher systemic risk premium on micro-level bank risk exposures. This would introduce a forward-looking approach to risk management that can be applied counter-cyclically to stabilize economic fluctuations.
In addition to the evidence from the U.S. financial sector, the authors show that the predictive power of CATFIN also remains strong for other countries. For example, the regional CATFIN can predict lower GDP growth of the Asian countries and the European Union six and eight months ahead, respectively.
CATFIN is part of a research agenda that covers asset pricing, behavioral finance, risk management, international finance, and corporate finance; moreover, it’s part of Tang’s interest in teasing out truths previously unrevealed by data analysis.
“I’m an empiricist. I have a genuine interest in most finance and economics questions that can be tested by data,” said Tang. “I focus primarily on asset pricing, and how people’s behavior can influence the outcome of market performance.”
Tang recently finished co-writing “Liquidity Shocks and Stock Market Reactions,” with Bali, Peng Lin, Ph.D., associate professor of economics and finance, and Yannan Shen, a doctoral candidate at Baruch College.
The paper was featured at the 2012 Chinese Finance Association’s Best Paper Symposium. It addresses a curious facet of liquidity, which refers to the degree to which a significant quantity can be traded within a short time frame without incurring a large transaction cost or adverse price impact.
A “liquidity shock” is any unexpected change in that transaction cost, be it a negative or positive change, which may be timed around new information about a company’s state.
While negative liquidity shocks usually lead to lower stock returns in the short term, Tang and his co-authors documented that, for firms with less investor attention, a negative liquidity shock will affect the stock price in a much slower manner than firms receiving more attention.
They also found that in certain circumstances, high liquidity shocks can predict a high return in the long term. But investors don’t take advantage of these predictions, because unlike earnings shocks, liquidity shocks are not something they pay close attention to or comprehend easily.
“Earnings shock is typically defined as the difference between actual earnings and analysts’ consensus, which is fairly simple to calculate and understand; investors can easily tell the difference between big and small earnings shocks,” he said. “It is, however, more challenging for them to quantify and interpret the pricing information embedded in liquidity shocks.”
Tang is now pursuing research into social networks and what they can tell investors.
“Users post their opinions about companies there, and investors want to understand whether or not these opinions actually convey useful information about future stock performance,” he said.
On the other hand, social media users may respond to corporate news in a biased way.
“If there is some sort of shock to the firm’s fundamentals, e.g., approval of a new drug by the FDA, we can use comments and opinions on social media to examine whether there exists systematic bias in interpreting these shocks,” Tang said.
“Maybe users comment less on positive but more on negative news, or vice versa,” he said. “And this generates a systematic bias in reacting to corporate news. Whether such bias has implications for future stock performance will be an interesting empirical question to explore.”