So much of the analog world has receded into memory, it’s hard to imagine there was a time when you had to drop film off at a store to have pictures developed or crack open a dictionary to look up the definition of a word. There’s even a currency—Bitcoin—that exists exclusively in the digital realm.
For Joshua Schrier, Ph.D., chemistry research is the next frontier.
“An emerging area of chemistry is finding ways to create a machine-readable representation of the things in the world, like the structures of molecules or chemical processes, and then using those digital representations for computer simulations and machine learning,” he said.
“Once we have the results of chemical experiments in a digital form, we can unleash the tools of data science to make smarter predictions. By combining this with robots that can conduct new experiments, we create the possibility for a virtuous cycle: Every new data point gives our model a better picture of the world, and algorithms can select new data points that improve that picture and dispatch experimental instructions to a robot to collect new data.”
Schrier joined the faculty in 2018 as the first Bepler Chair in Chemistry, and has devoted much of his time to his study “Discovering reactions and uncovering mechanisms of perovskite formation,” a $7.4 million project funded by the Defense Advanced Research Projects Agency.
Perovskites are a class of minerals that can be used in low-cost, high-performance solar cells, x-ray detectors, and lighting. The goal of the project is to develop software and hardware to automate scientific research, using perovskites as a test case.
Robot-Created Minerals
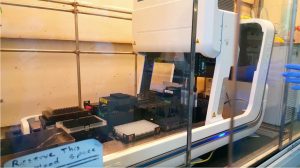
He and fellow researchers at Haverford College, Lawrence Berkeley National Laboratory, and MIT have developed a system dubbed RAPID (Robot-Accelerated Perovskite Investigation and Discovery) to create perovskite minerals. Perovskites are minerals composed of both inorganic and organic materials, which makes them are particularly attractive.
“You can replace the organic building unit with hundreds of thousands of different possible molecules. Every time you do that, you get a different crystal structure. It’s kind of like molecular Legos,” said Schrier.
“Our efforts are aimed at the early stage of materials development; we’re not making new solar cells themselves, but we are discovering the materials that will enable better solar cells. It’s like we’re not building a house, but we’re inventing new kinds of bricks you could use to build a house,” he said.
Exploring new structures is important, he said, because by changing the structure of the perovskites, you change the way they interact with light, their electrical properties, and their stability. This is important, he said, because one of the key limitations of existing perovskite solar cells is a lack of long-term stability.
In the three years since the project got underway, Schrier said they’ve synthesized roughly 70 perovskites and performed over 10,000 experiments. While that’s useful, he said, what’s equally important is that RAPID is learning how to do the experiments itself.
2020 Findings
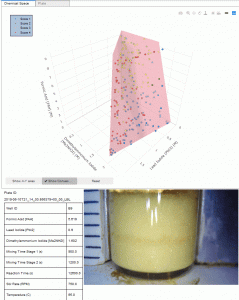
In “Robot-Accelerated Perovskite Investigation and Discovery,” an article published in June 2020 by Chemistry of Materials, he and his colleagues detail how they adapted perovskite syntheses for the RAPID system. Given a set of starting ingredients, researchers were able to conduct 96 randomly chosen experiments in four hours. That created a data set that the computer was able to then use to predict the success of future experiments.
Although he’s based in New York City and RAPID is housed at the Lawrence Berkeley National Laboratory, Schrier is able to work with colleagues in California remotely and his students are likewise able to analyze data safely from their homes. This paper was one of the top-20 most-downloaded papers in 2020, according to the journal.
This initial set of experiments is sufficient to predict the results of any subsequent experiments for that chemical system with 80-90% accuracy. In subsequent work published in the Journal of Physical Chemistry C, Schrier and co-authors Mary Kate Caucci, FCRH ’20; Michael Tynes, FCRH ’17, GSAS ’20; and Aaron Dharna, FCRH ’16, GSAS ’20, were able to show that researchers can also extrapolate to entirely new sets of chemical ingredients that have never been seen before, with about 40% accuracy.
“With no knowledge about this new chemical system, just the things that we’ve learned about in the past about other chemical systems, being right 40% of the time is good enough,” Schrier said. “This gives us a higher probability of success on our first batch of 96 experiments. We don’t need to be perfect, we only need to find one success. To use an analogy, machine learning lets us pick better lottery tickets, and the robot lets us buy more lottery tickets. Putting them together gives us the best chance of winning.”
Randomness and Removing Bias
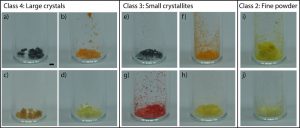
What’s surprised Schrier the most about recent findings is the effectiveness of randomness. Simply selecting the initial experiments randomly often yields better machine learning models than data chosen by human experts, he said.
This focus on randomness has important implications for artificial intelligence, because if human-generated data is used to create machine learning models, he said, we run the risk of creating machines that repeat our own biases. He explored the importance of removing human “fingerprints” in “Anthropogenic biases in chemical reaction data hinder exploratory inorganic synthesis,” which he published in 2019 in the journal Nature.
“This is at odds with the hypothesis-driven experiment design we teach students from grade school through university. What we’ve found is that humans tend to get stuck in a rut, and so instead of exploring all of the possibilities, they just focus on a few,” he said.
“The advantage of using robots is that they do what we tell them, even if it is just random. In this way, we remove our conceptual fingerprints from the data collection process and take a more unbiased look at the world.”
In the Classroom with Non-Science Majors
Although creating minerals from scratch is exciting, work with students is just as rewarding, Schrier said. In addition to mentoring six Fordham undergraduate research students, this fall, he taught a new course called Drug Discovery from Laboratory to the Clinic, which was especially fortuitous given the intense interest in the development of COVID-19 vaccines. The course is part of Fordham’s Manresa Scholars program and combines science with the Eloquentia Perfecta core.
Reading material for the class, which was for non-science majors, included analyses of Remdesivir, articles on clinical trials for hydroxychloroquine in the New England Journal of Medicine, analysis of the ethics of Moderna’s vaccine distribution plan, and information about the regulatory process of drug approval.
Outside speakers included a research scientist from the National Institute of Health and a pet-pharmaceutical startup entrepreneur who provided insights into the long path from basic research to sustainable business.
“The class just sort of wrote itself given the unfolding of world events that were occurring in the fall. The intersection of science, policy, business, and ethics is a fertile ground for engaging students,” he said.
“Fordham students have a rich intellectual toolbox for these types of discussions. In their core requirements, they’re taking philosophy, theology, economics, political science, and can apply this to the problem at hand. They’re quick to start a debate with, ‘No, no, no. Kant says you shouldn’t objectivize humans. We can’t do this.’”
Meanwhile, RAPID continues to churn out perovskites. Schrier is collaborating with Clavius Distinguished Professor of Computer Science“Mary Kate Caucci” “Michael Tynes” “Aaron Dharna” “Frank Hsu” “Yuanqing Tang”, to look at new ways of performing automated quality control for scientific experiments. He is also working with Rodolfo Keesey, FCRH ’20, in conducting data analysis geared toward using RAPID for other types of perovskite growth methods.
And in a collaboration with Fordham College at Rose Hill senior Lillian Cain and Michael Tynes that was published in a recent issue of the Journal of Chemical Education, Schrier described how algorithms for planning chemical experiments can be incorporated into a first-year general chemistry lab.
“We’re developing tools for doing science in a new way—not just perovskites—and it’s exciting to see Fordham students at the forefront of this new approach,” he said.