More Accurate Modeling is Critical, Fordham Economists Say
As the United States struggles to bring the COVID-19 pandemic under control, the race to create a vaccine is rivaled perhaps only by efforts to test everyone for the virus and deliver results in a timely manner.
According to a new study by Fordham economists, the government’s failure to provide widely available, timely, accurate testing has resulted in another problem. Because there are still people who have the virus but haven’t been tested, it has hobbled the government’s ability to accurately predict how many American will die from the virus in the future.
Testing Bias
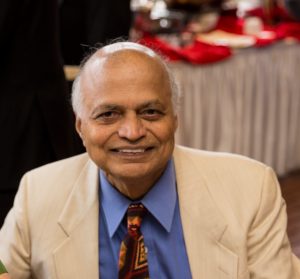
In Adjusted Bias-free Forecasts of Covid-19 Deaths for July 13 and July 20, a paper published on the Social Science Research Network on July 23, Hrishikesh D. Vinod, Ph.D., and doctoral student Katherine Theiss, shared the findings of a model that they say takes into account this skewed data, or “testing bias,” and makes a more accurate prediction of future deaths. (Update: Vinod and Theiss have published new forecasts with predictions through Election Day.)
Theiss and Vinod, a professor of economics and the director of Fordham’s Institute for Ethics and Economic Policy (IEEP), first published findings on the subject on July 7 in A Novel Solution to Biased Data in COVID-19 Incidence Studies. Adjusted Bias-free Forecasts is the fourth supplement to that publication, and it relies on what is known as an “autoregressive distributed lag model.”
“We are unaware of how many people truly have this infection, so that leads to issues when trying to predict disease outcomes,” said Theiss, who joined the economics doctoral program last year and is specializing in econometrics.
“If we’re trying to predict the amount of deaths in the future from the cumulative amount of Covid infections, what we’re currently doing is off, because we’re not tracking how many people actually have the infection. We only know what the tests are telling us.”
How Likely Is It That a Randomly Chosen Person Will Be Tested?
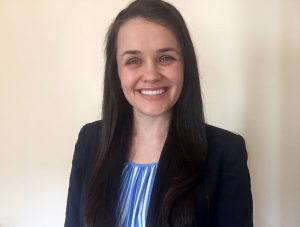
The paper rested on two equations. Since the country cannot test everyone yet, the first equation calculated the likelihood that a randomly chosen person in the United States will be tested for the virus. In the second equation, researchers used the results of the first equation to make predictions about what the future will look like.
To develop the first equation, they looked at socioeconomic and demographic variables, such as the share of hospital employees per capita in individual states, the percentage of residents who take public transit, the prevalence of hypertension, household income, and the percentage of residents who are uninsured.
Since data is not available for specific people, they generated simulated data for 500,000 individuals, and assigned each individual to a state based on the weighted probability of residence. To generate that probability, they took each state’s total population and divided it by the entire United States population.
Each simulated individual was assigned a testing index of 1 or 0 based on the weighted probability that they were tested for Covid-19 in their assigned state, which was in turn determined by taking the cumulative number of tests administered in each state and dividing it by the total state population.
Combined with socioeconomic, health, and demographic variables for the individual based on state of residence, they were able to develop a model that predicts the likelihood of a random person getting tested for Covid-19 on a statewide level.
High Testing Bias in Some States
The results, which change week to week based on statewide data, are not encouraging for some states. From the first equation, one can estimate the level of bias in state-level reporting of infections. In particular, states like Texas and Utah have stood out as having especially high levels of testing biases from April 20 to June 15. New York, by contrast, currently has one of the lowest biases, on account of its more robust testing regimen.
Theiss said variations between states are thought to be the result of the difference in time it took for states to implement mass testing strategies; New York has pushed relatively strong testing efforts since the initial outbreak, while some Southern states began later. Not surprisingly, those same states are seeing spikes in infections. The good news is that data shows a decrease in testing bias overall, which Thiess said points to improvements in testing administration and access.
Making Predictions After Adjusting for Bias
The second equation predicts future deaths from past infections after controlling for the bias associated with testing. The bias-adjusted model predicts future deaths in the United States more accurately than does the same model currently used by researchers, which is not corrected for testing bias. Using data through July 20, the unadjusted model underestimated U.S. deaths for the week ending July 27 by 5%. After correcting for the bias, the Fordham adjusted model overpredicted total deaths, but only by 1.6%.
County-Level Predictions Recommended
Vinod and Theiss use state-level data in their model to predict future nation-wide death count. While they plan to provide state-level predictions of deaths each week, they urge elected officials and public health professionals from each state to use their model to conduct similar analyses using county (census tract)-level data. By using local data, elected officials can obtain even more accurate state-level predictions of deaths, they said. In addition, their model could easily be used to predict alternative outcomes of interest, such as the demand for hospital beds and particular devices (e.g., ventilators).
Vinod and Theiss hope that their proposed methodology for predicting disease outcomes can help inform policy decisions.
“We believe that as we reopen and make these important decisions, we should take into account what’s going to happen in the future, and not only what’s happening now,” Theiss said.